- FR
- EN
The Power of Predictive Models
At SCOR we are working on model interpretability because we believe that we have a duty to provide every applicant with transparent risk assessments that provide them with a path forward to understand an outcome.
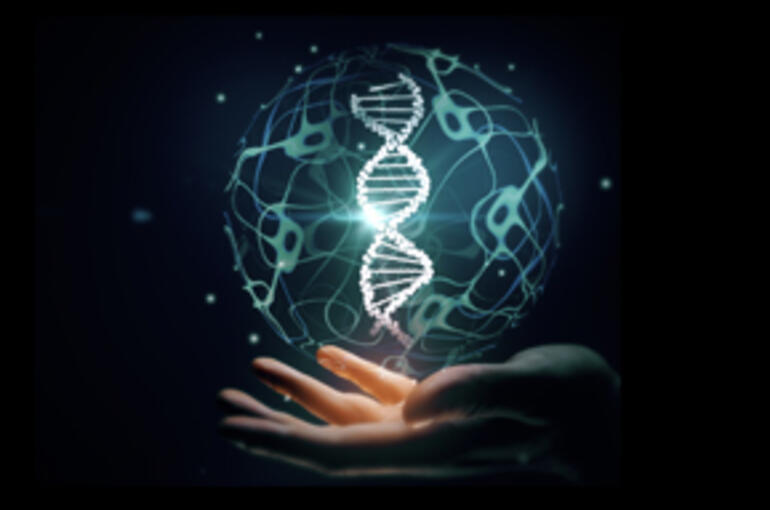
Predictive models are an incredibly powerful tool with the potential to drive the life insurance industry forward in ways that are good for both consumers (improving their purchasing experience by removing intrusive requirements and long delays) and carriers (increasing taken rates and persistency, and increasing the accuracy of mortality assessments). Traditional rules-based approaches start with assumptions and predict outcomes, while a predictive-model based strategy starts with outcomes and uses modelling techniques to identify data characteristics most likely to produce those outcomes. The predictive power of a model should be supported by a rules-based safety net.
This predictive power coupled with the continued emergence of new underwriting data sources, puts us on the cusp of a sea change in what it means to underwrite.
Even the state of NY Department of Financial services recognizes this, saying,“The Department fully supports innovation and the use of technology to improve access to financial services. Indeed, insurers’ use of external data sources has the potential to benefit insurers and consumers alike by simplifying and expediting life insurance sales and underwriting processes.”[1]
That same well-known Insurance Circular Letter No. 1 (2019) also comes with two important cautions:
- The data and processes that use it must not be unfairly discriminatory, and
- The underwriting outcome of any such data or process must include “the reason or reasons for any declination, limitation, rate differential or other adverse underwriting decision provided to the insured”.
These are reasonable requirements and completely consistent with the bar of social responsibility to which insurers are held. Transparency is exactly what is needed to help address these requirements.
Achieving that transparency can be a daunting obstacle to overcome for teams building predictive models. This challenge cannot be ignored.
As an industry we have the opportunity to use the power of Artificial Intelligence (AI) to provide insurance to offer peace of mind – helping individuals and families be more financially resilient to unexpected life events, enhancing our product offerings through innovation, ensuring alignment with changing consumer needs and extending insurance coverage beyond that provided by local social systems. Overcoming the transparency challenge is well worth the effort.
What is transparency?
There are two levels of transparency to consider in a modeling context. The first is transparency around how the model itself was built: what data went into it, how that data was collected and transformed, what elements of that data drive the predictions on an aggregate basis? Inspecting the model for bias throughout the build and refinement process should be a deliberate step in any model development lifecycle.
Involving subject matter experts on the underwriting process (the underwriters themselves) throughout the model development process is an important part of understanding where unintentional bias might be introduced. Underwriters are very familiar with this topic, as that concern is an important part of our current underwriting processes today. Data scientists and data engineers may not have enough context about the insurance domain to recognize where unfair discrimination might unintentionally be introduced.
The second level of transparency is just as important – and potentially more challenging to achieve. That is transparency at the individual prediction level (also known as “model interpretability”). For a given model outcome, can we explain why the model has reached that outcome?
Model interpretability is serving each individual in a way that can be understood by producers and applicants and can provide an applicant a path forward to dispute that outcome through required processes.
The Organization for Economic Co-operation and Development includes as part of its formal recommendation on the use of AI that “there should be transparency and responsible disclosure around AI systems to ensure that people understand AI-based outcomes and can challenge them.” [2]The existing processes around allowing an applicant to dispute their underwriting assessment is exactly the kind of use case to which that recommendation applies.
SCOR’s work
I’m excited about the industry-leading work we’re doing on model interpretability at SCOR. Our team has adopted a method of model introspection that allows us to clearly convey the driving variables behind model predictions as well as their directionality and relative impact.
To evaluate how important a feature is to a model and interpret what the model is doing we use Shapley additive explanations (SHAP). SHAP looks at how different a prediction would be with unknown information on a particular feature. It does so by incorporating both frequency and impact. We can use SHAP to understand the direction and magnitude that different values of features have on the prediction and to rank features according to their impact on the applicant's predicted outcome.
Figure 1 - Shapley additive explanations
The result of this introspection is included with the model output for every model we build – whether the model is designed to optimize your acceleration rate, refine your mortality stratification or detect potential anti-selection in your distribution channel. Each prediction will include clear reason codes and weights that support explainable, actionable next steps that can be used in any step of the underwriting process and in any state in the country.
We need to put the days of “black box” models behind us and commit as an industry to transparency both in model design and in the predictions those models provide. Failure to do so will result in these powerful tools being eliminated from our toolbox.
About the author
Al Mele, SVP, Underwriting Innovation at SCOR, manages a multi-disciplined team that is a driving force behind the company’s value-added services strategy. An employee since 1999, Al began his career at SCOR as a programmer/analyst working on reinsurance administration systems. His knowledge, leadership and focus on client service contributed to his success and earned him more challenging roles within the organization.
Prior to his current role, Al led SCOR’s Velogica team, which offers an innovative web-based underwriting solution. Al’s history with Velogica began as a technical lead overseeing the development of the tool in 2004 and over the years has included operations, IT and business development responsibilities.
Al earned a Bachelor of Science degree with distinction in mathematics from the University of North Carolina at Chapel Hill.
[1] https://www.dfs.ny.gov/industry_guidance/circular_letters/cl2019_01
[2] http://www.oecd.org/finance/The-Impact-Big-Data-AI-Insurance-Sector.pdf