- FR
- EN
Insurtechs are using digital infrastructure to deliver the products of tomorrow
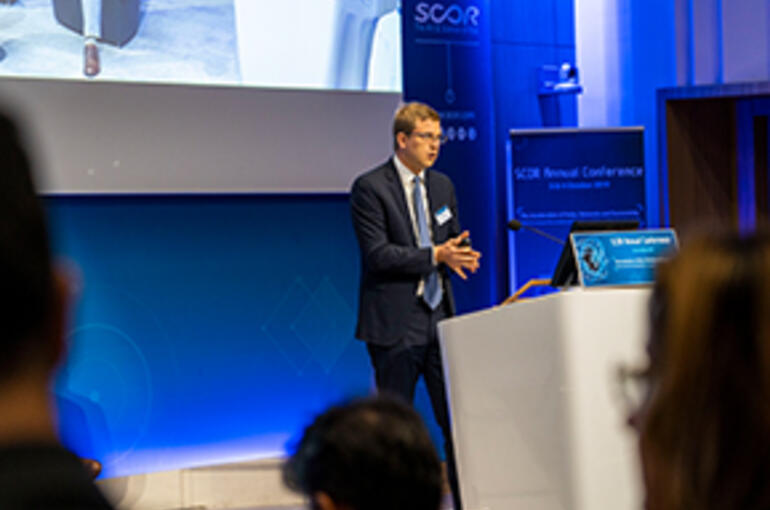
At the 2019 SCOR Annual Conference, Adrian Jones, Deputy CEO of SCOR P&C Partners, spoke with the heads of data science from two SCOR partners: Andy Mahdavi of States Title and Arnaud Alepee of Hokodo.
States Title uses machine intelligence to make residential real estate closings simpler and more efficient. Hokodo makes insurance and financing available to millions of European SMEs who are not well-served by today’s financial services industry.
Although the word InsurTech is barely five years old, InsurTech is as old as insurance. In 1867, a land surveyor named Daniel Sanborn started making highly detailed maps of every city and town in the US, which soon became a standard tool for insurance underwriting. The standard pricing policy included pulling out a Sanborn map book, finding an address, measuring the distance to a fire hydrant.
The basic concept is similar to how business is done today, 150 years later, and yet the way we do it is entirely different. Every 20 years, a technology has greatly altered the way insurers operate. In the 1950s, it was the mainframe computer, which enabled large multi-line insurers to develop, operating big parts of an insurer on a single computer. The minicomputer came in the 1970s, with greater flexibility to enable specialist underwriters to flourish. The personal computer and spreadsheet in the 1990s enabled sophisticated risk modelling at one’s fingertips, which was critical for catastrophe underwriting and risk management.
Today, a number of powerful technologies have come together around data infrastructure to allow the rise of InsurTech – cloud-based storage and processing, extensive new data sets, mobile, social, geospatial analytics.
SCOR forms partnerships with leaders in using new technology. We believe that these technologies will first be differentiators and soon become table stakes for successful insurers and reinsurers. As with previous waves, today’s technologies will transform the industry, but more slowly than the hype would lead you to believe.
I asked Andy and Arnaud, to share how their firms are using data infrastructure.
Andy Mahdavi: what is your focus at States Title?
States Title’s goal is to make residential real estate closings vastly simpler and more efficient through the use of technology. The process of buying and selling a home in many parts of the US is coordinated by a title company. The title company is responsible for validating and insuring that the buyer has the rights to the property described in the deed, as described in the deed. Title defects range from unpaid tax bills to multiple parties claiming ownership of the same property.
In some European countries, a notary or government agency transfers title, guaranteeing a buyer a clean title or recourse if there are problems. In the US, property deeds and encumbrances are filed at county recorders’ offices, who stamp the documents as having been presented for recording but do not validate their correctness. It’s a “buyer beware” situation: the buyer must search the recorder’s records to identify anyone with a prior claim on the property, and the buyer must immediately record their own ownership to avoid claims by others to own the land. Hence title companies.
Over the years, title has become a concentrated business in the US, with four companies controlling over 90% of it. They run at an incredibly low 4% loss ratio, but expense ratios are typically in the 80s or low 90s. These high expense ratios are the result of investing heavily in sales and operations that require costly, time-intensive, manual processes. To date, title companies have been focused on automation to increase human productivity. States Title is the first title company to apply machine intelligence to eliminate low-value-added human work and focus humans on the exceptions and difficult situations that require human intervention.
The real estate data infrastructure in the US has become quite robust over the past 20 years. High quality land records and transaction data are available in many jurisdictions. Our algorithm uses state-of-the-art machine learning techniques to ingest all the available property information starting with only an address.
From the data we ingest, we can directly observe many title defects and predict the risk of unidentified defects eventually affecting a property. We set a confidence threshold such that we instantly underwrite 80% or more of title refinance policies. The remaining 20% is sent to humans via traditional underwriting approaches, with the machine having identified why it believes issues exit. Tests by our customers have shown that the model is highly robust at identifying title issues that require manual review or resolution.
Thus, we have a balanced approach. We use machine intelligence where machines perform well and deliver the best value to the customer. We use humans, informed by machines, where a machine cannot yet.
Arnaud Alepee: what is the role of machine learning at Hokodo?
Our focus is single-invoice trade credit insurance. Studies show that in the UK, 30% of bankruptcies are caused by the nonpayment of an invoice. But traditionally, only large companies have had access to invoice insurance. We saw a need and a market for providing trade credit insurance to small businesses at the invoice level.
We saw three main challenges:
- We needed to automate the underwriting process, since there was no way we could afford to review every invoice one by one.
- We needed to price it in real time, which means assessing the risk of someone not paying an invoice.
- We needed to distribute the high acquisition costs.
Application Programming Interface (API) provided the solution and is at the core of everything we do. Explained succinctly, an API is like a message protocol between systems or companies, like Uber using Google maps. Basically, you need five pieces of information to insure an invoice:
- Who is the client?
- Who is the client dealing with?
- What is the amount of the invoice?
- What is the issue date?
- What is the due date?
Machine learning gathers all this data. Then we push it into algorithms that map customers and give us the risk probability. Machine learning and digital products allow us to feed the results back to the user in real time to let them know if their invoices are protected. In this process, partnership is fundamental. I benefit from my partners’ customer base and I offer them my API.
At SCOR we are building the insurers (and reinsurers) of tomorrow. We provide our risk capacity, our expertise, and sometimes investments. Ultimately, we believe that leveraging the emerging data infrastructure will make the insurance industry itself better able to serve its purpose of efficiently protecting people, businesses, and governments from losses.